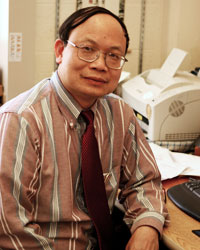
Prof. Sun-Yuan Kung
Princeton University, USA
Title: X-Learning: Bootstrapping BP (Back-Propagation) with BB (Back-Broadcast) for Joint Parameter/Structural Learning of Deep Networks
Abstract: While deep learning has by and large dominated the field of machine learning, curse of depth and lack of structural learning remains to be two formidable challenges to the prevailing Back-Propagation (BP) learning paradigm, which recursively compute the gradients of a given External Optimization Metric (EOM). Our solution to the curse-of-depth lies in Back-Broadcast (BB) of teacher values, a dual approach to Forward-Skip in ResNet. Complementary to ResNet’s input-residual learning, BB supports an output-residual learning - a process resembling innovation learning in estimation theory. On the other hand, the challenge on structural learning warrants some profound and rigorous mathematical foundations: (a) The structural gradients are often derived from a pre-specified Local Optimization Metric (LOM), aka Structural Optimization Metric (SOM). Our LOM is based on a Discriminant Information (DI) stemming from a combination of Fisher’s discriminant analysis and Shannon’s mutual information. (b) Such structural gradients help point to precisely which neurons in a hidden layer should be removed in order to optimize the LOM. (These neurons are termed deleterious neurons: DNs.) More rigorously, LOM rises when and only when DNs are removed during structural pruning. The rising LOM score in structural learning will then in turn bootstrap the EOM score in parameter learning. (c) The proposed DI also boasts a close consistency between the LOM (for structural optimization) and EOM (for parameter optimization). This plays a vital role in X-learning, an Net-Parameter (NP) Iterative Learning paradigm. Thanks to such consistency, DI-based LOM and EOM will effectively bootstrap each other during NP-iterations to to jointly optimize the parameters/structure of the CNNs. Theoretically, there exists a useful equivalence between maximizing DI versus minimizing LSE. In practice, it implies that X-learning can find applications in both the classification and regression scenarios. We shall demonstrate that X-learning has indeed yielded performances superior to previous winners of competition in low-power ImageNet classification and winners of super-resolution challenge on PIRM imaging systems. To further showcase its versatility, we shall show how X-learning may be successfully deployed for regression-classification hybrid systems, which represents a novel and promising application paradigm.
Bio: S.Y. Kung, Life Fellow of IEEE, is a Professor at Department of Electrical Engineering in Princeton University. His research areas include machine learning, data mining, systematic design of (deep-learning) neural networks, statistical estimation, VLSI array processors, signal and multimedia information processing, and most recently compressive privacy. He was a founding member of several Technical Committees (TC) of the IEEE Signal Processing Society. He was elected to Fellow in 1988 and served as a Member of the Board of Governors of the IEEE Signal Processing Society (1989-1991). He was a recipient of IEEE Signal Processing Society's Technical Achievement Award for the contributions on "parallel processing and neural network algorithms for signal processing" (1992); a Distinguished Lecturer of IEEE Signal Processing Society (1994); a recipient of IEEE Signal Processing Society's Best Paper Award for his publication on principal component neural networks (1996); and a recipient of the IEEE Third Millennium Medal (2000). Since 1990, he has been the Editor-In-Chief of the Journal of VLSI Signal Processing Systems. He served as the first Associate Editor in VLSI Area (1984) and the first Associate Editor in Neural Network (1991) for the IEEE Transactions on Signal Processing. He has authored and co-authored more than 500 technical publications and numerous textbooks including "VLSI Array Processors", Prentice-Hall (1988); "Digital Neural Networks", Prentice-Hall (1993) ; "Principal Component Neural Networks", John-Wiley (1996); "Biometric Authentication: A Machine Learning Approach", Prentice-Hall (2004); and "Kernel Methods and Machine Learning”, Cambridge University Press (2014).
2nd Keynote Speaker
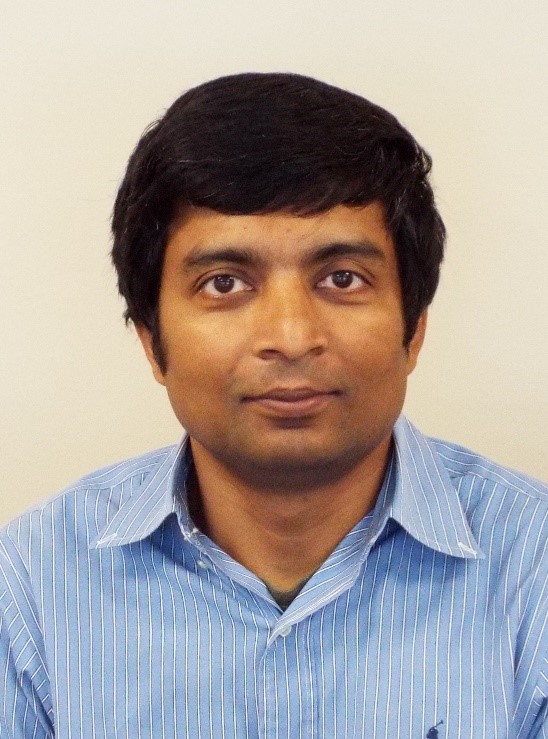
Prof. Latifur Khan
University of Texas at Dallas, USA
Topic: Big Data Stream Analytics and Its Applications
Abstract: Political event data record interactions among social and political actors. Researchers use these data to understand relations among actors, predict outcomes of interest, and forecast trends. As automated technologies have become better able to extract events from text, event data projects and repositories have increased in number. The main goal of this tutorial is to integrate and expand our end-to-end cyberinfrastructure for robust creation, validation, access, and analysis of political event data. We focus on political and social events about conflict and cooperation between governments, individuals, non-governmental organizations, rebel groups, and others. Natural language processing tools along with ontologies/dictionaries will be utilized to code event data by annotating the kinds of political events. In the talk we will show how to scrape contemporaneous news reports in English and Spanish, and automatically encode relevant political events for data analysts. Multiple challenges will be presented and addressed them in the talk: (1) additional extensions of multilingual framework with additional languages and types of events; (2) smoother updates to political actor dictionaries; (3) robust data querying and linking mechanisms, and analytic tools for the broader research community; and (4) improved methods for focus location extraction across languages and resolutions. This is a collaborative work with political scientists, Dr. Patrick Brandt and Dr. Jennifer Holmes, funded by NSF.
Bio: Dr. Latifur Khan is currently a full Professor (tenured) in the Computer Science department at the University of Texas at Dallas, USA where he has been teaching and conducting research since September 2000. He received his Ph.D. degree in Computer Science from the University of Southern California (USC) in August of 2000. Dr. Khan obtained his B.Sc. degree in Computer Science and Engineering from Bangladesh University of Engineering and Technology (BUET), Dhaka, Bangladesh in November of 1993 with First class Honors. He was a recipient of Chancellor Awards from the President of Bangladesh. Dr. Khan is an ACM Distinguished Scientist and received IEEE Big Data Security Senior Research Award, in May 2019, and Fellow of SIRI (Society of Information Reuse and Integration) award in Aug, 2018. He has received prestigious awards including the IEEE Technical Achievement Award for Intelligence and Security Informatics and IBM Faculty Award (research) 2016. Dr. Latifur Khan has published over 300 papers in premier journals such as VLDB, Journal of Web Semantics, IEEE TDKE, IEEE TDSC, IEEE TSMC, and AI Research and in prestigious conferences such as AAAI, IJCAI, CIKM, ICDE, ACM GIS, IEEE ICDM, IEEE BigData, ECML/PKDD, PAKDD, ACM Multimedia, ACM WWW, ICWC, ACM SACMAT, IEEE ICSC, IEEE Cloud and INFOCOM. He has been invited to give keynotes and invited talks at a number of conferences hosted by IEEE and ACM. In addition, he has conducted tutorial sessions in prominent conferences such as SIGKDD 2017, 2016, IJCAI 2017, AAAI 2017, SDM 2017, PAKDD 2011 & 2012, DASFAA 2012, ACM WWW 2005, MIS2005, and DASFAA 2007. Currently, Dr. Khan’s research area focuses on big data management and analytics, data mining and its application over cyber security, complex data management including geo-spatial data and multimedia data. His research has been supported by grants from NSF, the Air Force Office of Scientific Research (AFOSR), DOE, NSA, IBM and HPE. More details can be found at: www.utdallas.edu/~lkhan/